Introduction to LLM
Total of 17 articles available.
Currently on page 1 of 1.
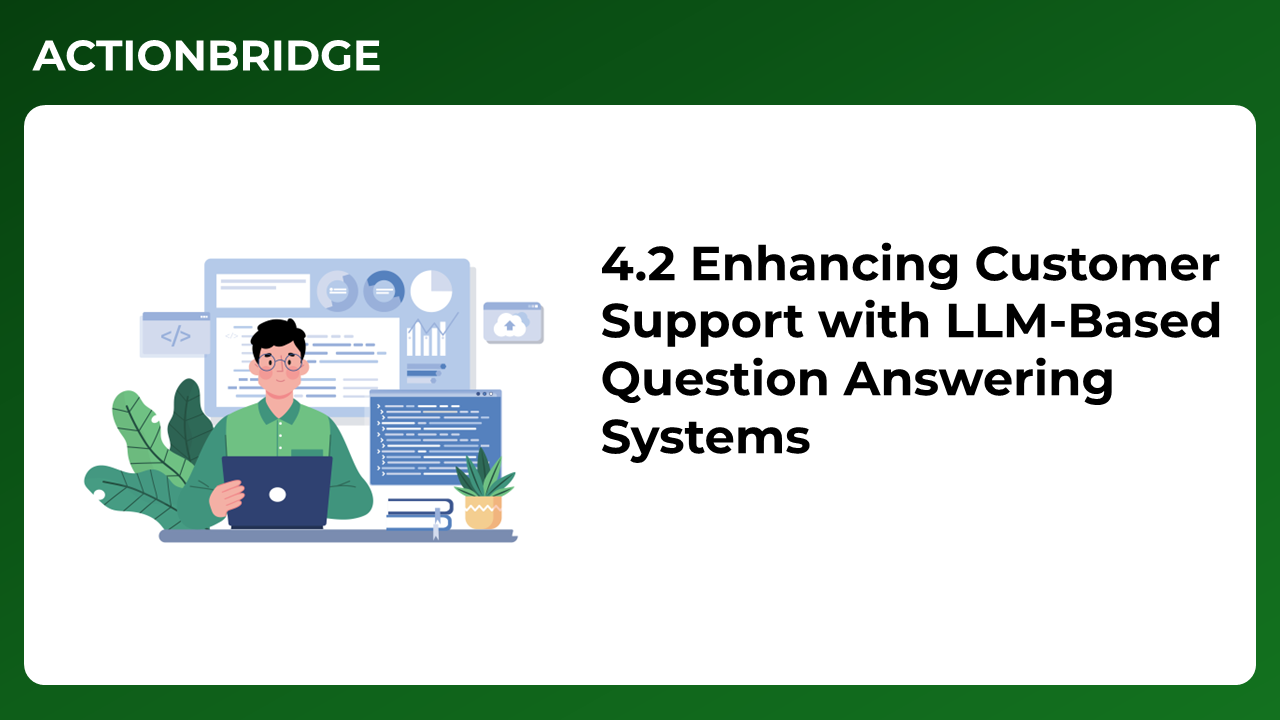
4.2 Enhancing Customer Support with LLM-Based Question Answering Systems
Discover how Question Answering Systems powered by Large Language Models (LLMs) are transforming customer support, search engines, and specialized fields with high accuracy and flexibility.
2024-09-25
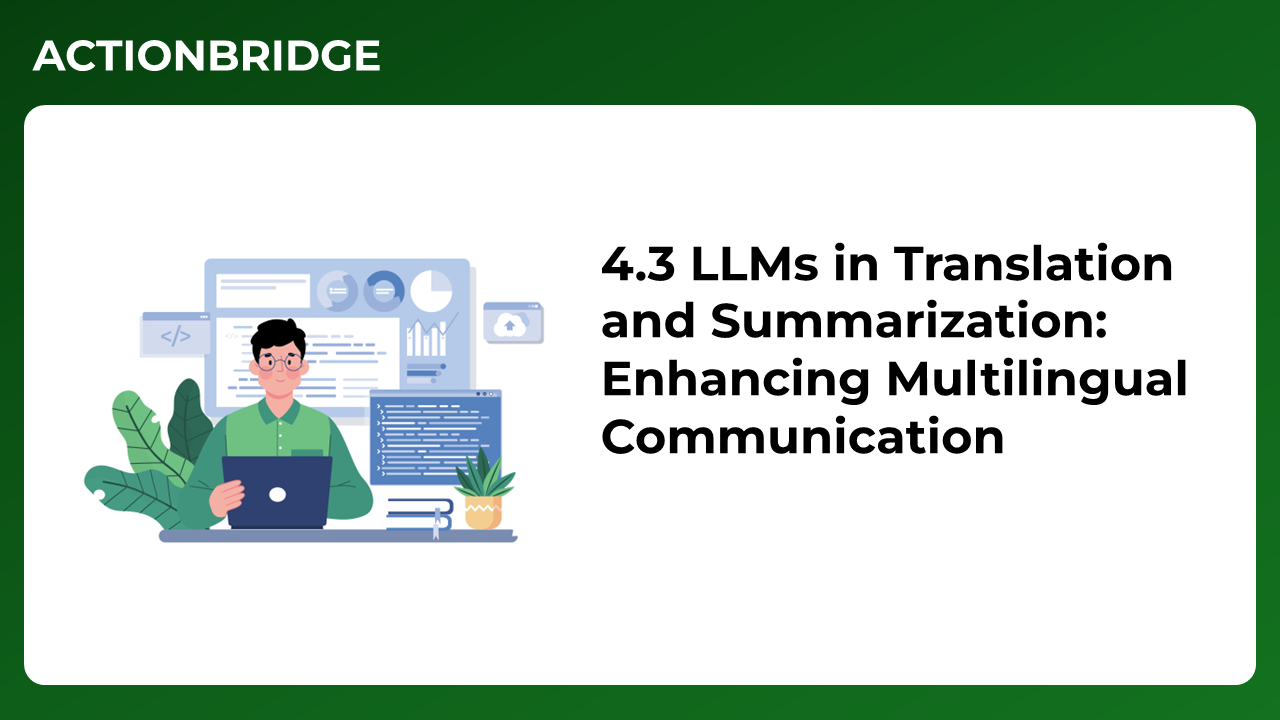
4.3 LLMs in Translation and Summarization: Enhancing Multilingual Communication
Learn how Large Language Models (LLMs) leverage Transformer architectures for accurate translation and summarization, improving efficiency in business, media, and education.
2024-09-18
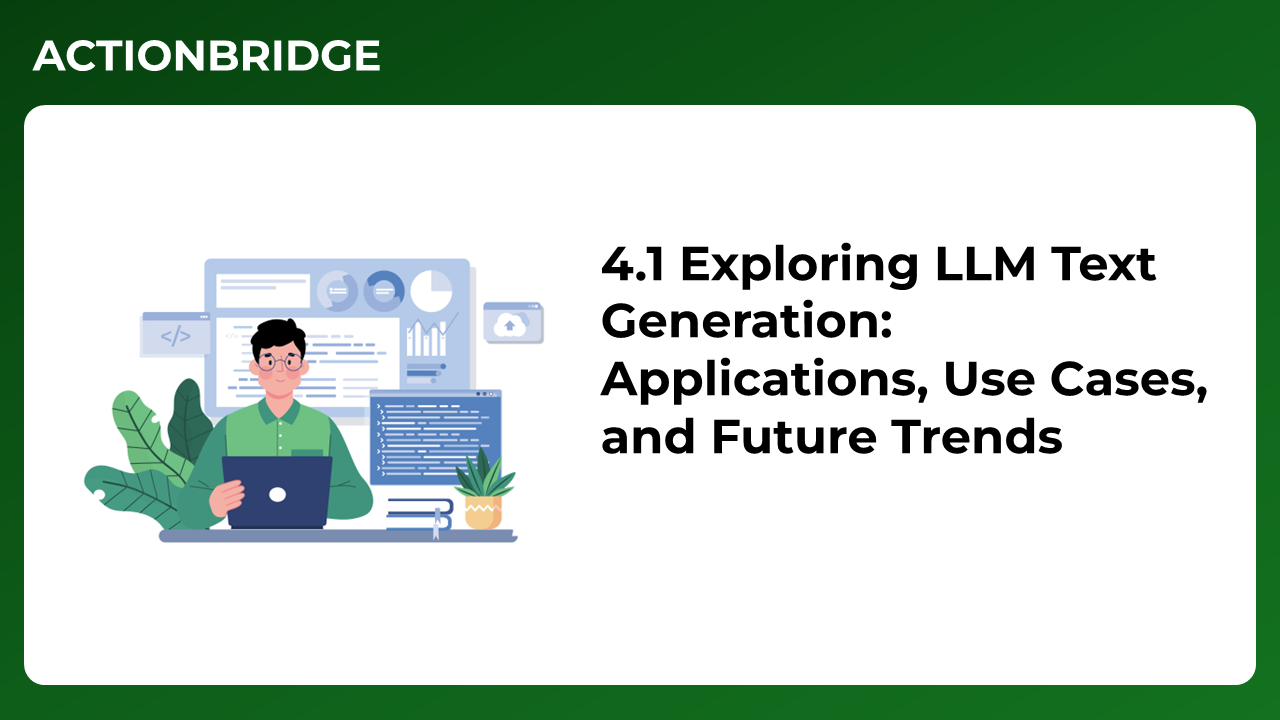
4.1 Exploring LLM Text Generation: Applications, Use Cases, and Future Trends
Learn how Large Language Models (LLMs) are applied in text generation for content creation, email drafting, creative writing, and chatbots. Discover the mechanics behind text generation and its real-world applications.
2024-09-16
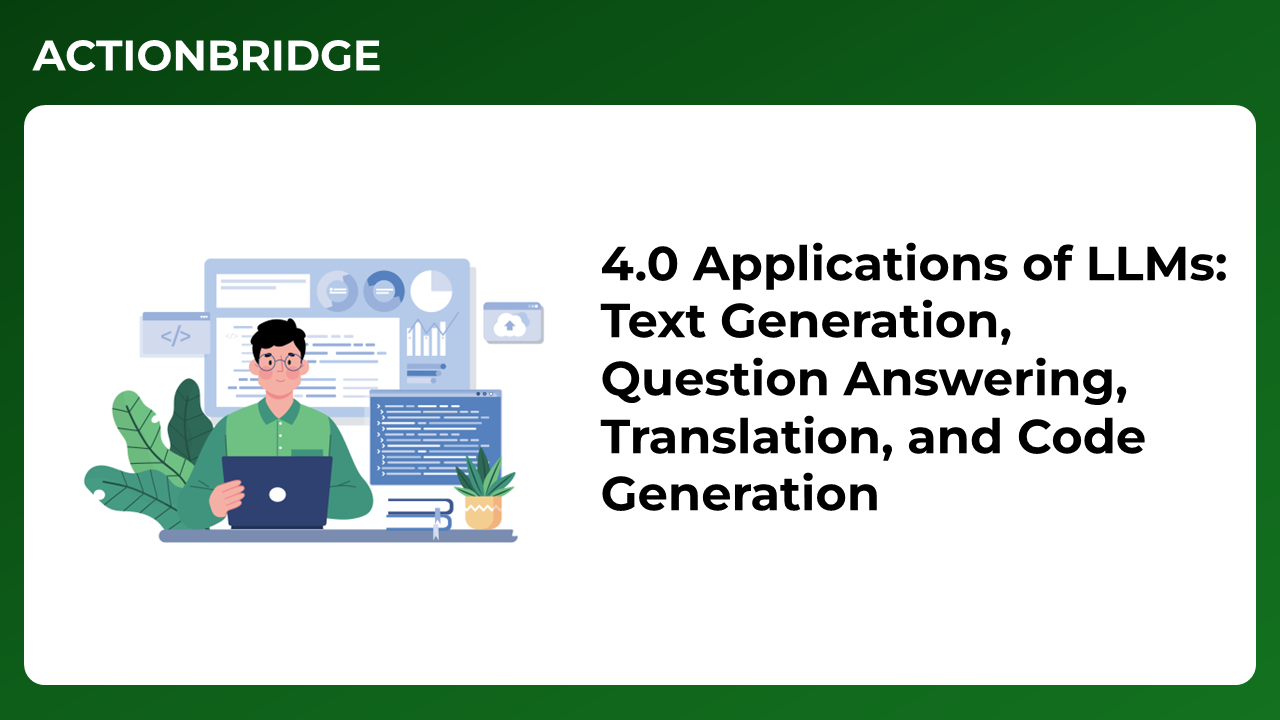
4.0 Applications of LLMs: Text Generation, Question Answering, Translation, and Code Generation
Discover how Large Language Models (LLMs) are used across various NLP tasks, including text generation, question answering, translation, and code generation. Learn about their practical applications and benefits.
2024-09-15
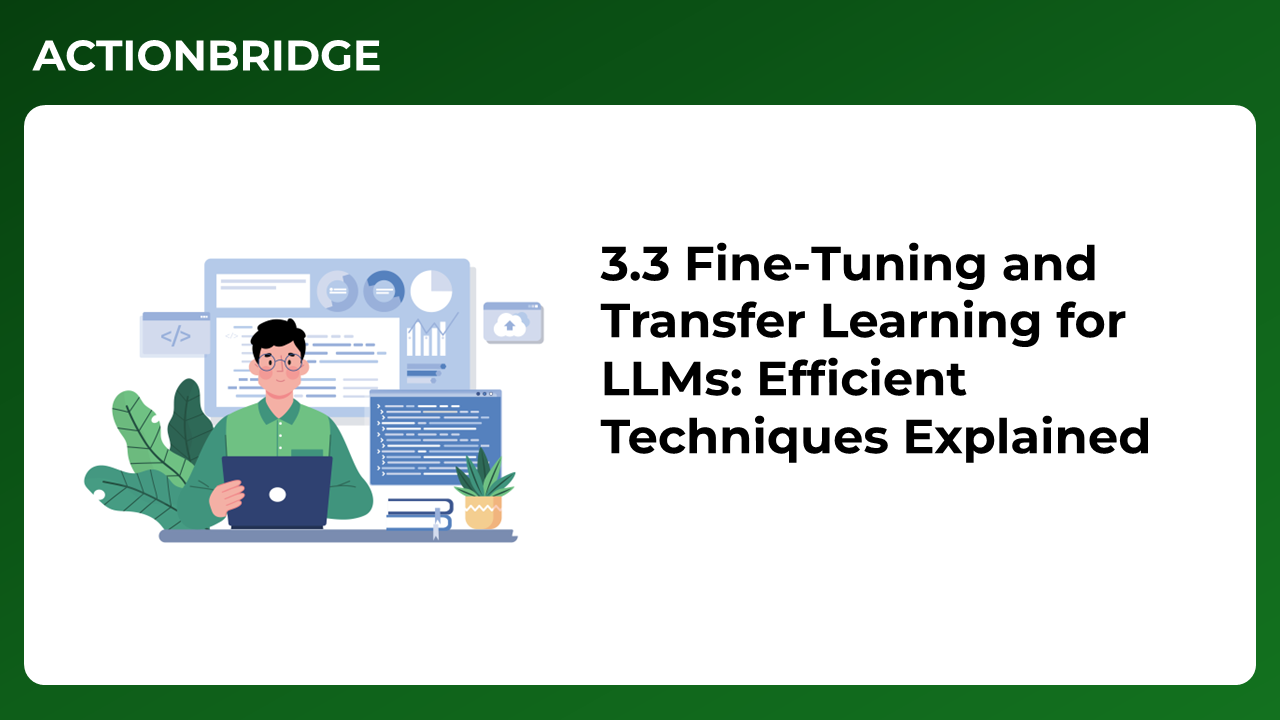
3.3 Fine-Tuning and Transfer Learning for LLMs: Efficient Techniques Explained
Learn how fine-tuning and transfer learning techniques can adapt pre-trained Large Language Models (LLMs) to specific tasks efficiently, saving time and resources while improving accuracy.
2024-09-14
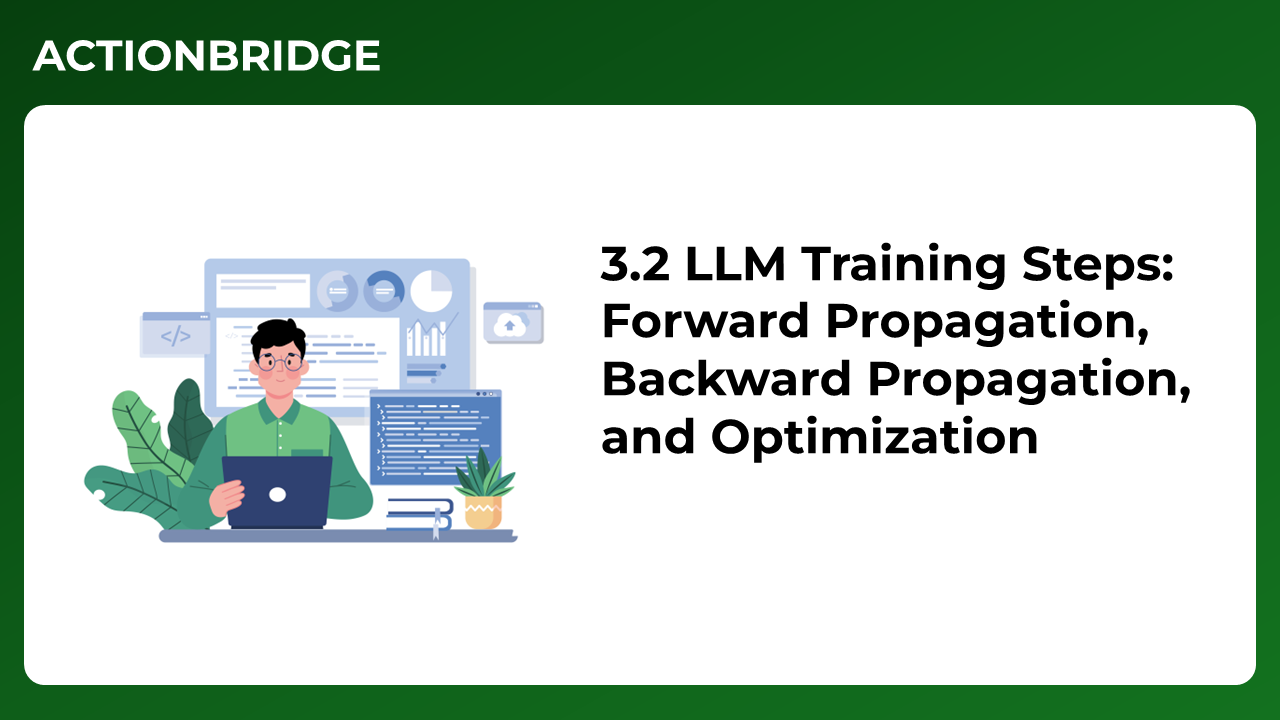
3.2 LLM Training Steps: Forward Propagation, Backward Propagation, and Optimization
Explore the key steps in training Large Language Models (LLMs), including initialization, forward propagation, loss calculation, backward propagation, and hyperparameter tuning. Learn how these processes help optimize model performance.
2024-09-13
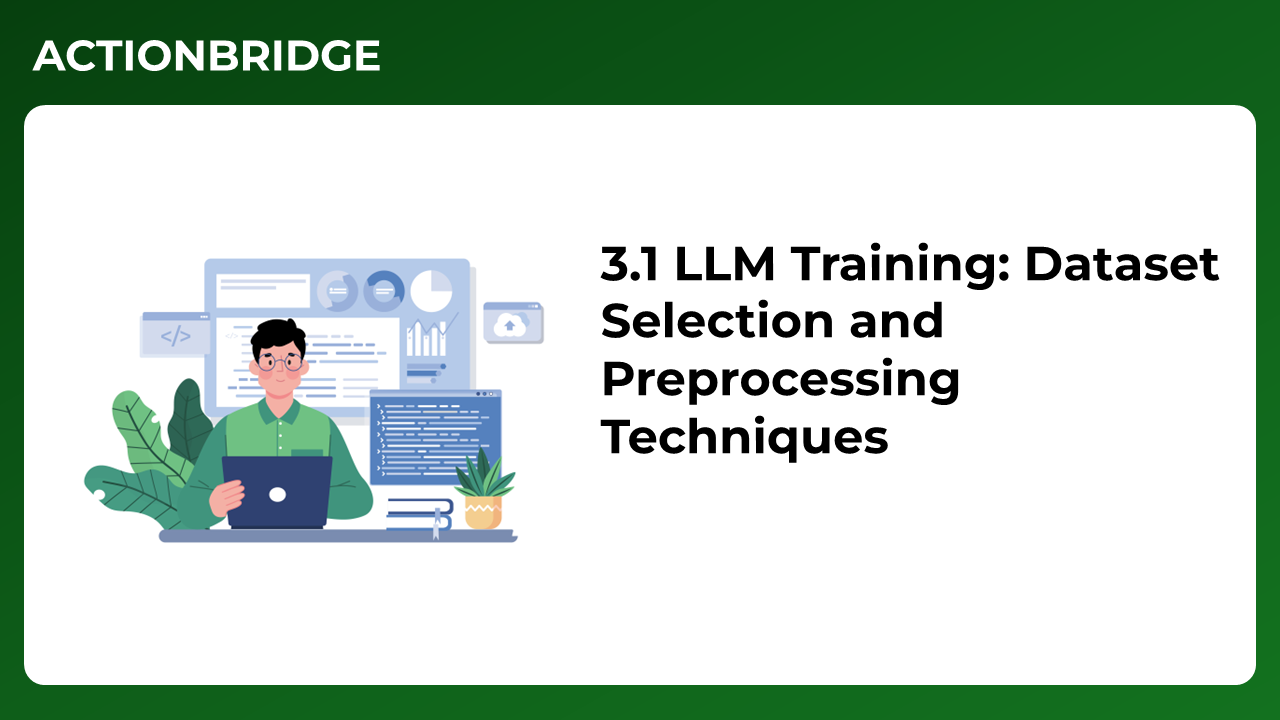
3.1 LLM Training: Dataset Selection and Preprocessing Techniques
Learn about dataset selection and preprocessing techniques for training Large Language Models (LLMs). Explore steps like noise removal, tokenization, normalization, and data balancing for optimized model performance.
2024-09-12
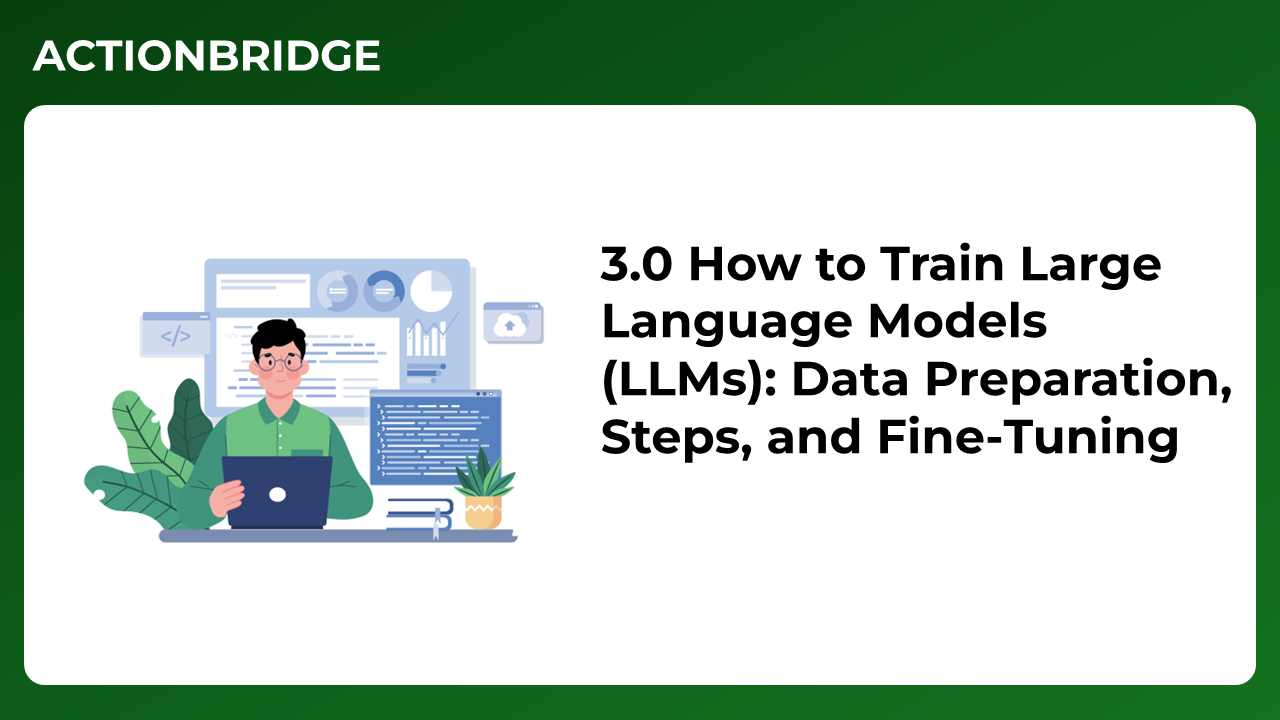
3.0 How to Train Large Language Models (LLMs): Data Preparation, Steps, and Fine-Tuning
Learn the key techniques for training Large Language Models (LLMs), including data preprocessing, forward and backward propagation, fine-tuning, and transfer learning. Optimize your model’s performance with efficient training methods.
2024-09-11
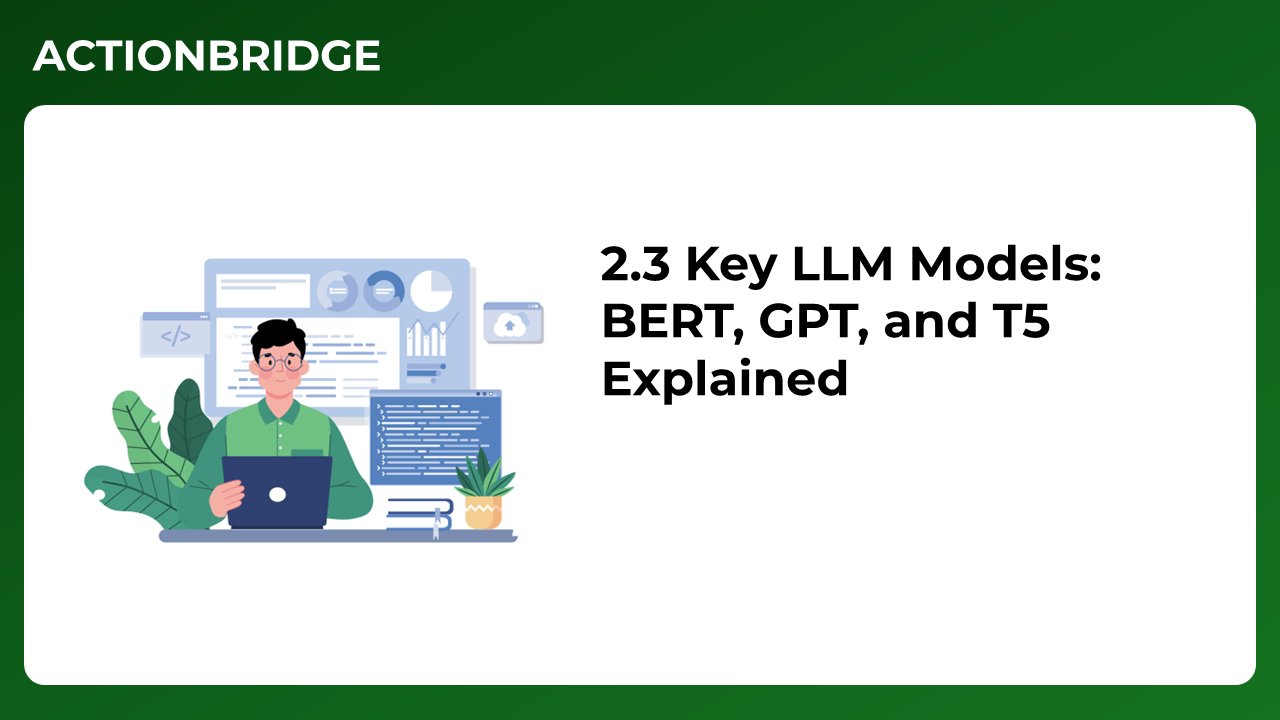
2.3 Key LLM Models: BERT, GPT, and T5 Explained
Discover the main differences between BERT, GPT, and T5 in the realm of Large Language Models (LLMs). Learn about their unique features, applications, and how they contribute to various NLP tasks.
2024-09-10
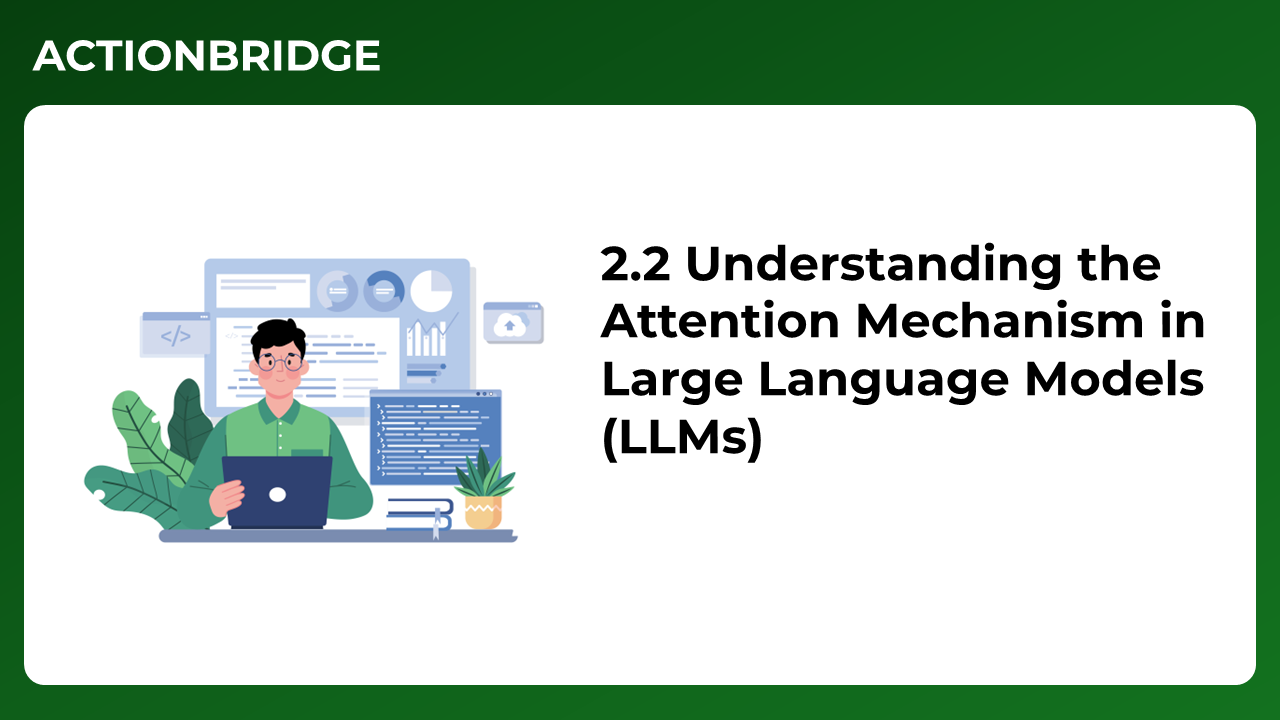
2.2 Understanding the Attention Mechanism in Large Language Models (LLMs)
Learn about the core attention mechanism that powers Large Language Models (LLMs). Discover the concepts of self-attention, scaled dot-product attention, and multi-head attention, and how they contribute to NLP tasks.
2024-09-09
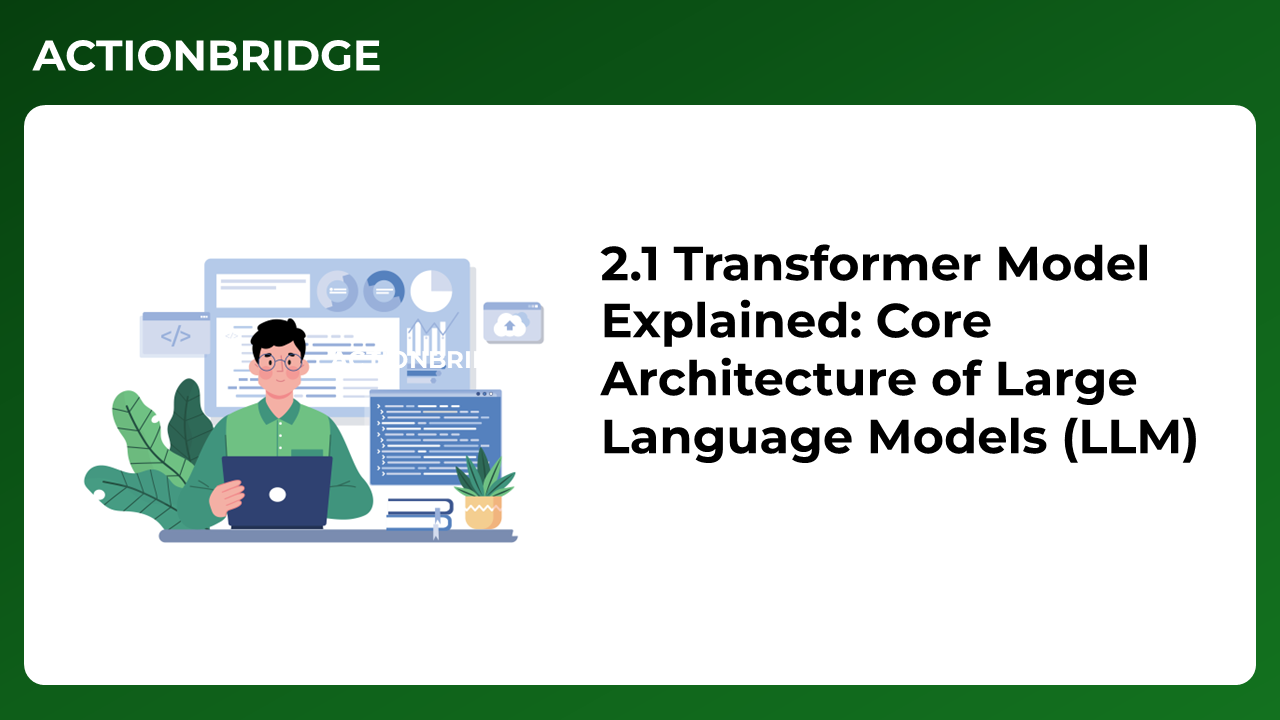
2.1 Transformer Model Explained: Core Architecture of Large Language Models (LLM)
Discover the Transformer model, the backbone of modern Large Language Models (LLM) like GPT and BERT. Learn about its efficient encoder-decoder architecture, self-attention mechanism, and how it revolutionized Natural Language Processing (NLP).
2024-09-07
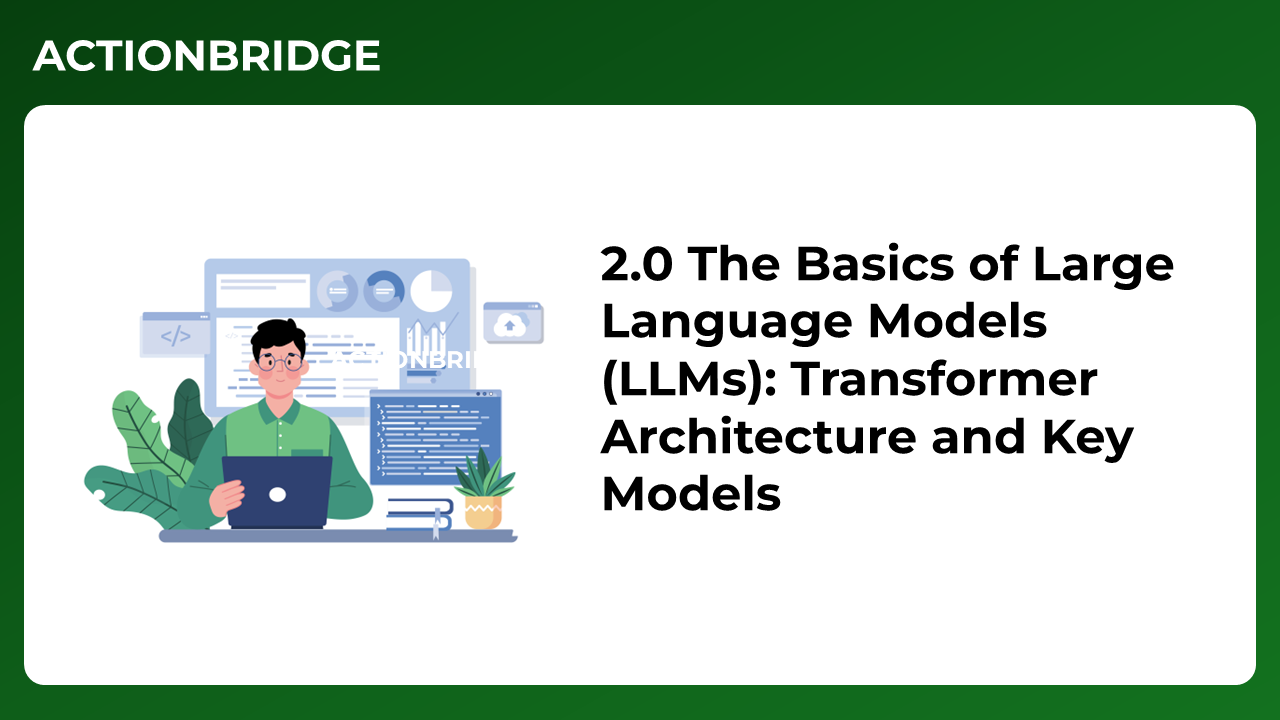
2.0 The Basics of Large Language Models (LLMs): Transformer Architecture and Key Models
Learn about the foundational elements of Large Language Models (LLMs), including the transformer architecture and attention mechanism. Explore key LLMs like BERT, GPT, and T5, and their applications in NLP.
2024-09-06
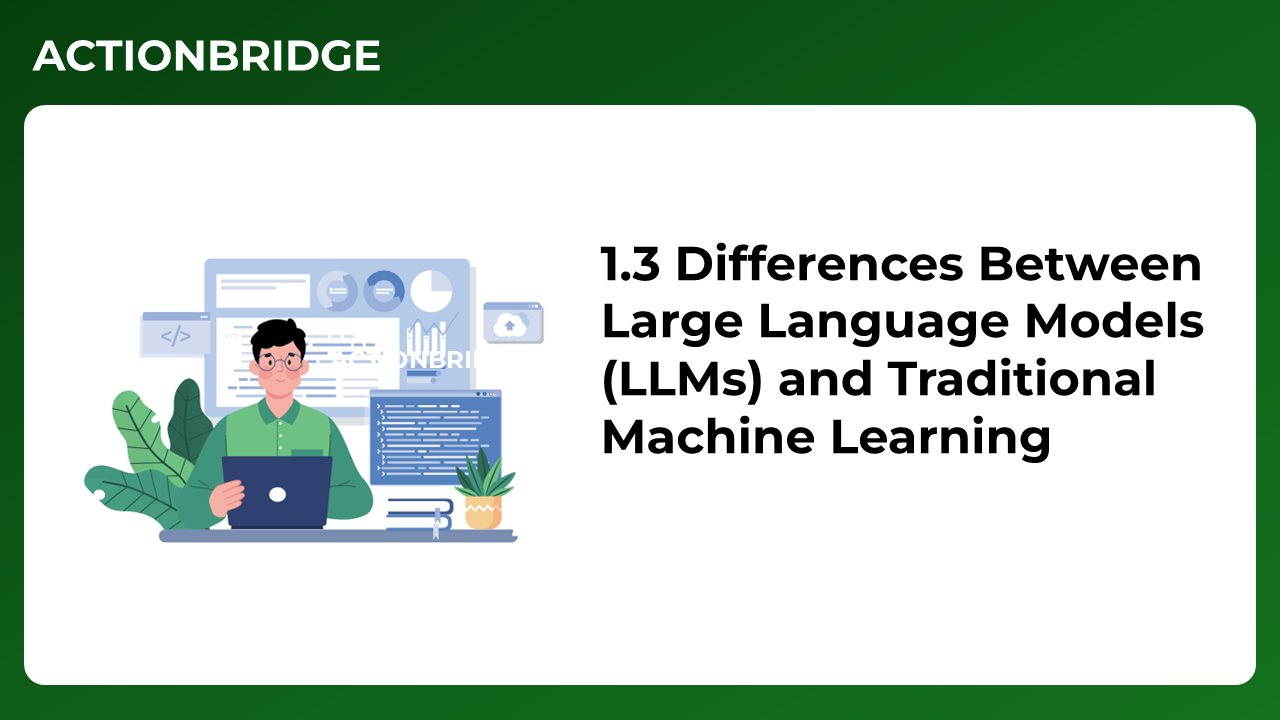
1.3 Differences Between Large Language Models (LLMs) and Traditional Machine Learning
Understand the key differences between Large Language Models (LLMs) and traditional machine learning models. Explore how LLMs utilize transformer architecture, offer scalability, and leverage transfer learning for versatile NLP tasks.
2024-09-05
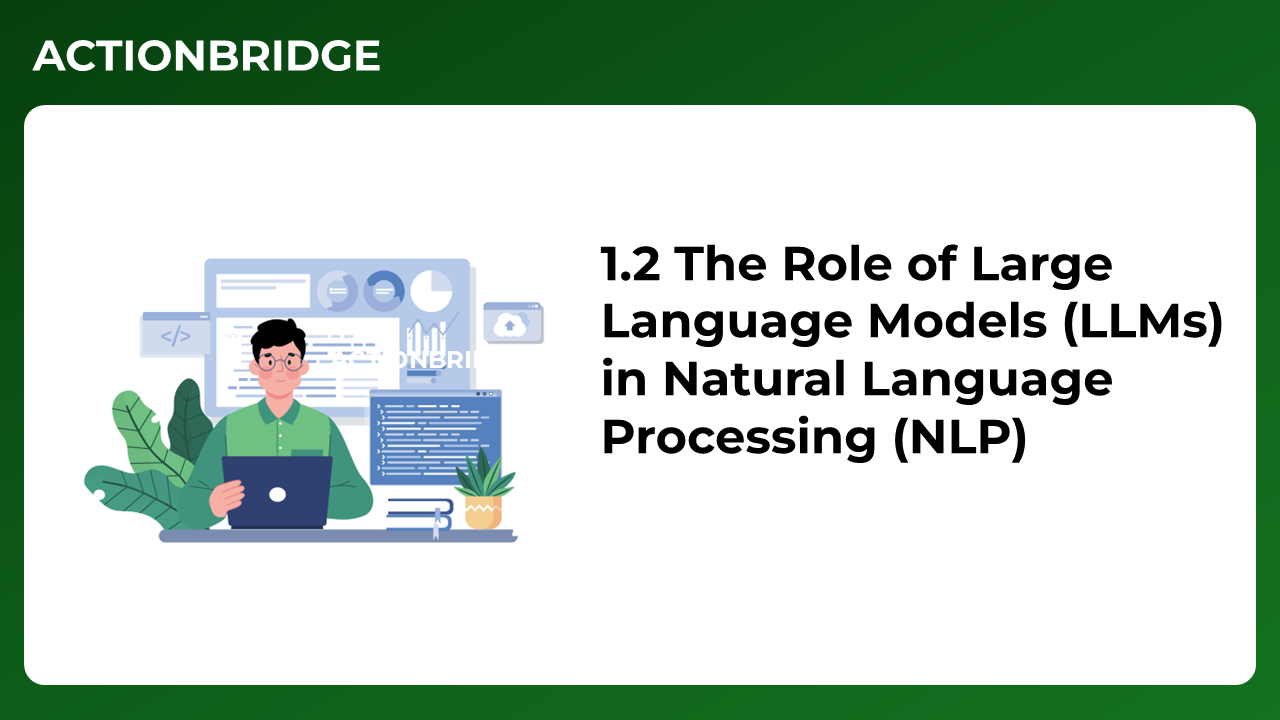
1.2 The Role of Large Language Models (LLMs) in Natural Language Processing (NLP)
Discover the impact of Large Language Models (LLMs) on natural language processing tasks. Learn how LLMs excel in text generation, question answering, translation, summarization, and even code generation.
2024-09-04
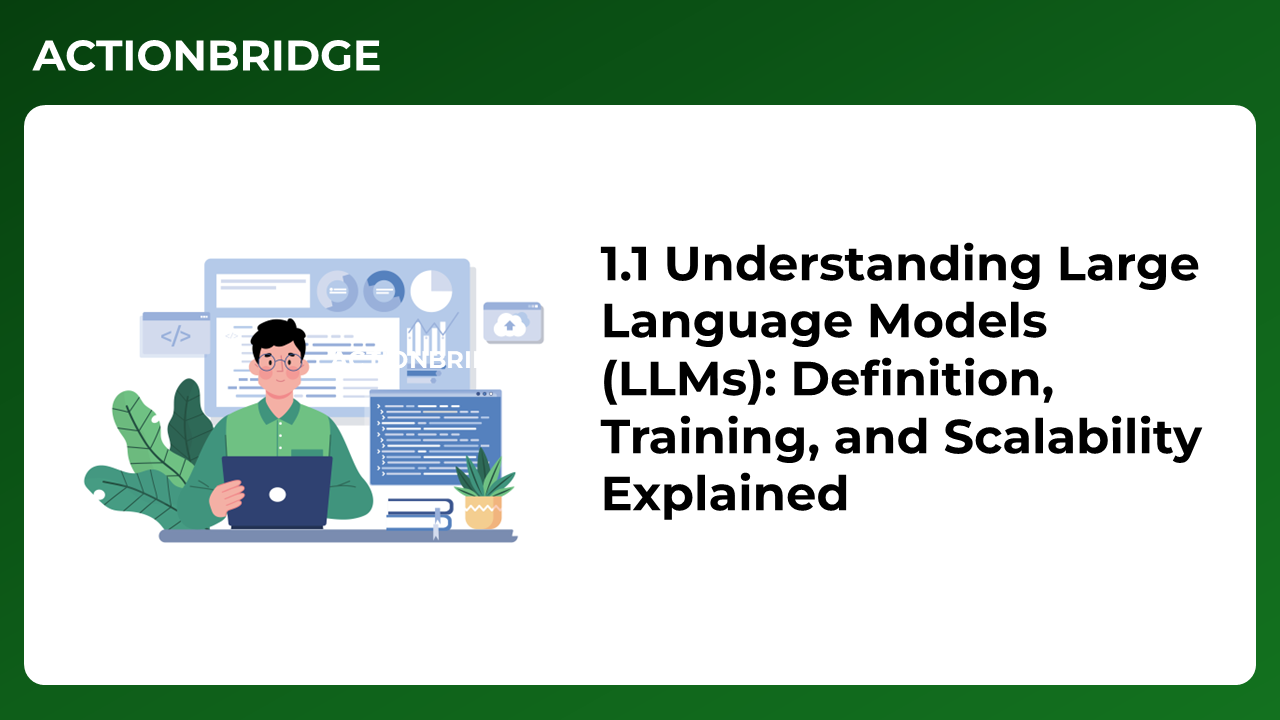
1.1 Understanding Large Language Models (LLMs): Definition, Training, and Scalability Explained
Explore the fundamentals of Large Language Models (LLMs), including their structure, training techniques like pre-training and fine-tuning, and the importance of scalability. Discover how LLMs like GPT and BERT work to perform NLP tasks like text generation and translation.
2024-09-03
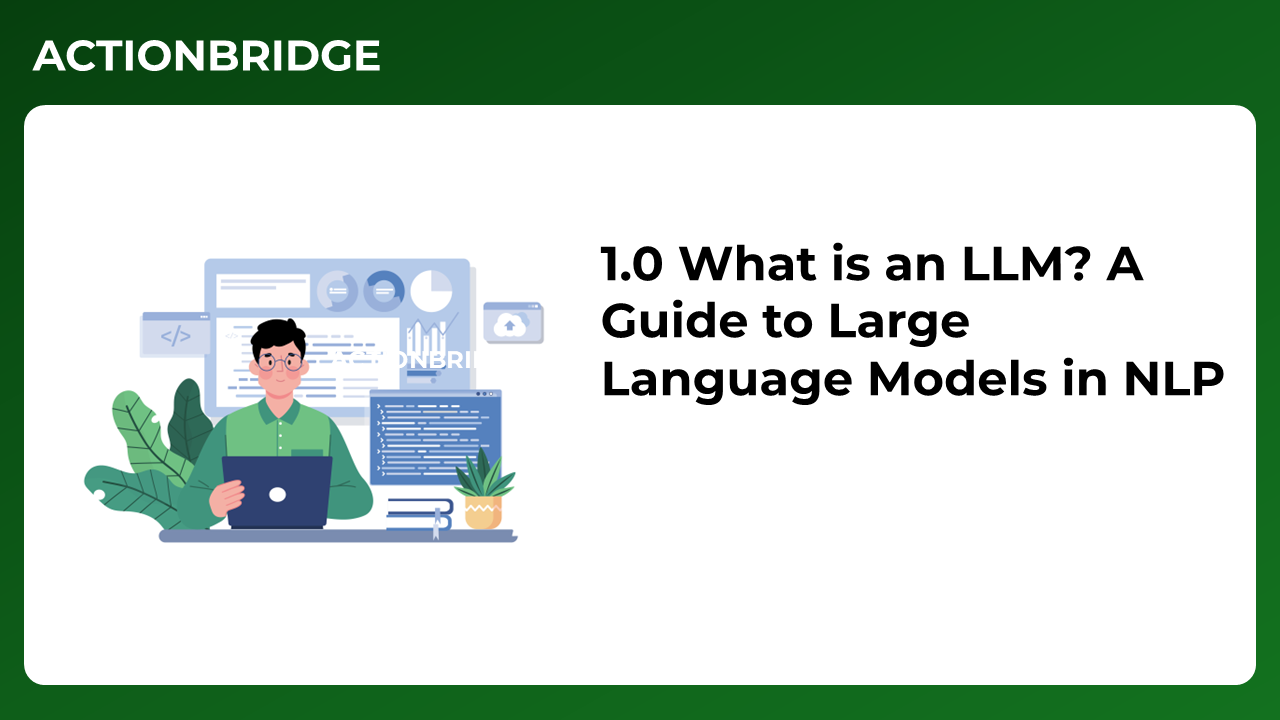
1.0 What is an LLM? A Guide to Large Language Models in NLP
Discover the basics of Large Language Models (LLMs) in natural language processing (NLP). Learn how LLMs like GPT and BERT are trained, their roles, and how they differ from traditional machine learning models.
2024-09-02
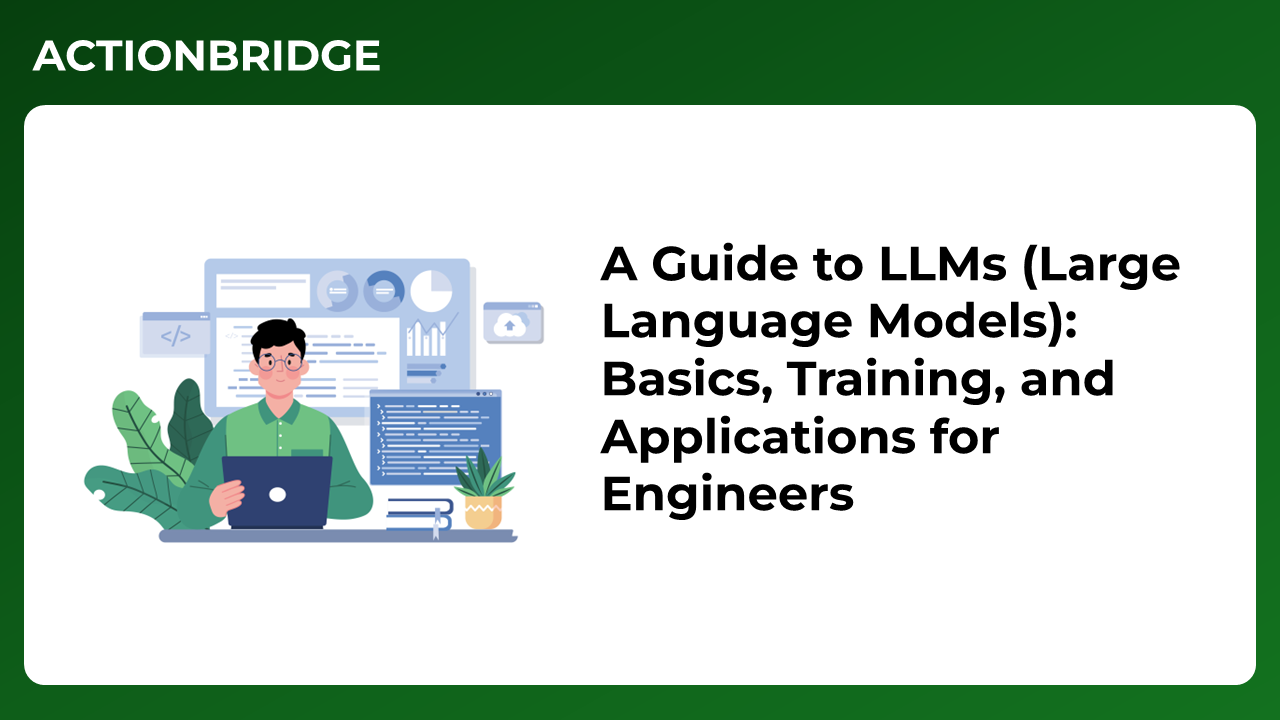
A Guide to LLMs (Large Language Models): Basics, Training, and Applications for Engineers
Learn about large language models (LLMs), including GPT, BERT, and T5, their functionality, training processes, and practical applications in NLP. This guide provides insights for engineers interested in leveraging LLMs in various fields.
2024-09-01
Category
Tags
Search History
améliorations 618
interface do usuário 609
modèles de tâches 606
Produktivität 594
búsqueda de tareas 579
colaboración 576
atualizações 558
interfaz de usuario 538
2FA 533
AI-powered solutions 507
language support 497
Aufgaben suchen 495
Aufgabenverwaltung 480
feedback automation 477
ActionBridge 476
joindre des fichiers 476
Version 1.1.0 457
busca de tarefas 448
Aufgabenmanagement 445
new features 444
modelos de tarefas 439
Teamaufgaben 438
anexar arquivos 434
Transformer 432
interface utilisateur 431
mentions feature 407
Google Maps review integration 394
customer data 392
CS data analysis 387
Two-Factor Authentication 380
Authors
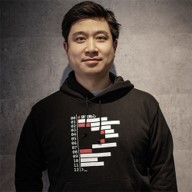
SHO
As the CEO and CTO of Receipt Roller Inc., I lead the development of innovative solutions like our digital receipt service and the ACTIONBRIDGE system, which transforms conversations into actionable tasks. With a programming career spanning back to 1996, I remain passionate about coding and creating technologies that simplify and enhance daily life.